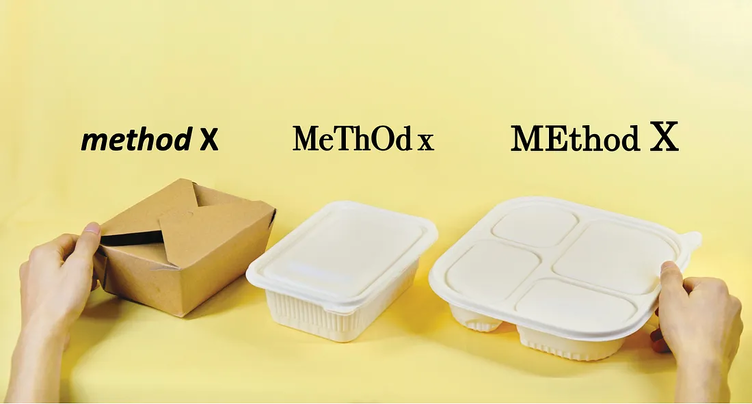
In the past, intellectual thieves were relatively straightforward and would simply copy well-written phrases from other works, sometimes from multiple sources, to create a publication. However, plagiarism has evolved over time, taking on various forms [1], and there have been famous cases of plagiarism [2].
As digital technologies advanced, simple text comparisons became capable of easily detecting plagiarized text, even when it was deceptively modified. In response, thieves shifted their focus to plagiarizing ideas. Oxford University defines plagiarism for its students as “Presenting work or ideas from another source as your own, with or without consent of the original author, by incorporating it into your work without full acknowledgement” [3]. However, the concept of “full acknowledgement” can be open to interpretation.
Who plagiarizes ideas is required to formulate the idea using his own words, displaying a level of intelligence to disguise the theft. By omitting citations to the work of their victim, the thieves claim the idea as their own. Although these cases are more complex, they can still be detected through time stamps and track records, allowing the original creator to be identified.
In today’s AI-driven era, researchers face immense pressure to publish rapidly and frequently. The phrase “Publish or Perish” has evolved into “Publish Fast or Perish Soon.” This pressure has driven some researchers to engage in unethical practices such as data fabrication and the omission of crucial details in order to gain recognition [4, 5]. Both cheaters and thieves operate in an environment with lowered ethical standards, driven by the intense pressure to succeed.
The Era of RePackaging?
In recent years, plagiarism detection technology has significantly improved [6]. It has become more challenging to simply steal intellectual property, leading many clever plagiarizers, especially in lucrative fields like data science and AI, to adopt a different approach known as “repackaging.” Repackaging can be considered a more sophisticated form of plagiarism.
To successfully repackage an idea, algorithm, or deep model, one must have expertise in the field. One needs to possess knowledge of the terminology and be familiar with “uncommon” methods that can be used to create the illusion of novelty during the repackaging process. Essentially, the core ideas or original algorithm or processing chain are taken, and new components that are relatively uncommon are added. This is then combined with a level of ambiguity and a multitude of results to create a repackaged work. To further enhance its appeal, the repackaged work is given a new name and, if the individual is particularly savvy, a flashy and captivating acronym. In this way, a paper with a “new” idea that appears superior to others is created, allowing the repackager to claim ownership over it.
However, one crucial aspect is that the original work must be cited, preventing accusations of plagiarism from both humans and plagiarism-detection software. The repackagers can defend themselves by saying, “I have cited your work, haven’t I?”
Prerequisites for Repackaging Ideas
To successfully execute repackaging, several other factors come into play. Firstly, it is important to note that repackaging typically occurs in a downward direction and not in an upward direction. This means that individuals with lesser-known or unknown affiliations cannot effectively repackage the ideas of those affiliated with prestigious institutions such as Ivy League schools or large corporations with extensive research activities. These institutions and individuals are often powerful, organized, and capable of taking legal action against cases of upward repackaging. Publishers are more likely to believe the claim that the average individual has stolen ideas from a more accomplished individual, making the chances of success for upward repackaging highly unlikely.
Downward repackaging, on the other hand, involves a researcher or team of researchers with an excellent affiliation attempting to steal ideas from individuals associated with less prestigious institutions. In both cases, the terms “excellence” and “mediocrity” refer to the institutions rather than the individuals themselves. The individual from a lower-ranked institution who engages in repackaging is likely to fail as their assertions are not generally believed. Conversely, the individual from a higher-ranked institution who repackages ideas from a lower-ranked institution is more likely to succeed as their claims are more readily accepted.
It is common knowledge that the ranking and status of one’s affiliation can significantly impact their career. When it comes to publications, institutional bias or favouritism is a recognized but challenging phenomenon to detect and objectively measure [7, 8]. Bias within the publishing industry can take various forms [9]. Elite schools with established fame and reputation often benefit from favouritism and biased evaluations [10, 11, 12].
The motivation behind repackaging ideas from less prestigious sources is not simply a matter of intelligence or competence. It involves a combination of factors. Firstly, there are overambitious researchers, both students and professors, who are eager to publish groundbreaking works in the field of AI. These ambitious authors may not have a strong understanding of the nuances of academic integrity. However, it is expected that a seasoned advisor with a high level of academic integrity would guide and teach them the ethical principles of conducting research. The presence of a young and inexperienced advisor, who is perhaps more distinctly driven to publish, can contribute to a lax approach to academic honesty. In such cases, the advisor may not effectively enforce ethical standards and may be more willing to compromise on integrity for the sake of achieving ambitious research goals. This combination of an overambitious student and an inexperienced advisor within a prestigious institution may create an environment conducive to repackaging ideas. This scenario is often observed because researchers at elite schools and institutions face heightened pressure to be both creative and productive. The pervasive “publish or perish” culture amplifies the pressure on these individuals, making them more susceptible to ethical lapses as they strive to meet the high expectations placed upon them.
However, despite these concerns, it is important to note that dishonesty, even in complex forms such as repackaging, will eventually be identified by the peer review system. Senior scientists often acknowledge this fact. Nevertheless, the peer review system is not without its flaws. Improperly selected reviewers may fail to detect insidiously repackaged ideas, and biased journals may prioritize articles from higher-ranked institutions in order to enhance their own reputation. It can be seen as advantageous for an editor when a majority of articles in their journal originate from prestigious institutions. This issue may become even more significant when dealing with editors who receive a salary for their position. While professors generally take on editorial tasks voluntarily for the betterment of the field and their own reputation, editors who are financially dependent on the quality of the journal may be inclined to prioritize acquiring “excellent” articles, leading to a perception that articles from prestigious institutions automatically equate to exceptional research. If the chief editor lacks AI expertise, the environment becomes conducive to the acceptance of repackaged work; most likely smart repackagers do select journals of general reputations but with no special competency in the filed pertinent to the repackaged work.
Yes, repackaged ideas can get published. Whereas conventional plagiarism may still be done and easily get detected [13], smart repackaging is hard to see through.
An Example
Below an example (that I know firsthand) to demonstrate how complicated things can get, and how difficult it may be to spot the repackaging.
- Original paper: Yottixel image search engine [14] introduces a novel Divide & Conquer approach for large histopathology image search. The “Divide” phase involves a multistage clustering technique based on staining/proximity, followed by guided sampling within the clusters. On the other hand, the “Conquer” phase utilizes a DenseNet architecture, followed by feature binarization and matching (see Figure 1).
Yottixel is published in a journal with established track record in medical image analysis, particularly in image search (1200+ hits when searched for retrieval/CBIR/search), and its editors are experts in the field. - Repackaged work: Another method, called SISH [15], utilizes the same Divide technique as Yottixel, which is already a major red flag for repackaging. This is why the admission that SISH uses Yottixel’s Divide has been obscured within the paper’s convoluted descriptions. Additionally, SISH employs the same DenseNet and binarization as Yottixel. Thus far, SISH is essentially equivalent to Yottixel. Of course, the repackagers must introduce some modifications to justify claiming ownership of the repackaged model. Consequently, SISH incorporates an autoencoder and a tree for discrete features in parallel with DenseNet and binary values. SISH represents a slightly expanded version of Yottixel (see Figure 1) but is repackaged as a new method.
SISH is published in a journal with no established reputation in medical image analysis (less than 200 hits when searched for retrieval/CBIR/search; zero for CBIR), and its editor has no track record in AI or in medical image analysis.
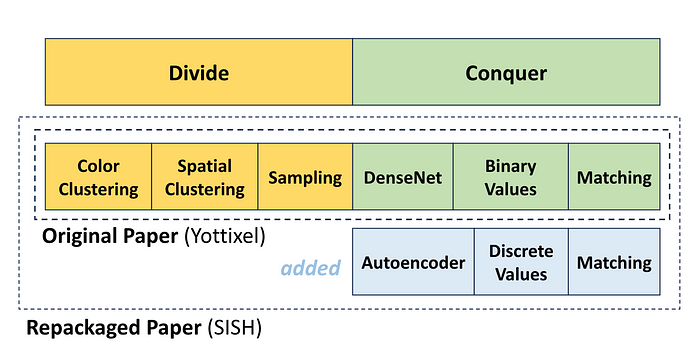
One may examine the authors’ affiliations of original and repackaged works, in conjunction with the competencies of the journal editors, to gain more insight. However, as repackaging of a complex AI paper often has intricacies, the interested reader may have to read both papers in detail and any comments (e.g., comments by Yottixel’s authors [16]) before making any conclusive evaluation.
Is repackaging plagiarism?
Does a repackaged paper constitute plagiarism? Technically, no.
Based on conventional definitions repackaging is not plagiarism because the repackagers cite their sources and do not copy/paste text from the original work. However, successful repackagers may, intended or not, steal credits and merits from the original authors and inventors by redirecting attention from the original work to the repackaged paper, which is largely not their own. In this sense, repackaging constitutes a new type of plagiarism that aims at pirating credit and reputation by reselling existing ideas under a different name but citing the plagiarized works to get away with the theft.
The Main Damage of Repackaging
Of course the authors of any original work may see themselves as victims of an insidious theft, and this is most likely true in most repackaging cases. But this is not the main damage that the “smart” repackagers inflict. The most pressing question is not even why major journals with high reputation would publish such works. The actual question is rather why we, as scientists, are driven to steal each other’s credit.
In the era of fast-paced AI competition, we have become ‘publication machines’ obsessed with sensational news, where every single paper must be presented as a breakthrough to impress our employers for sake of promotions and recognitions. Many papers are rejected at major AI conferences for lacking novelty. Our institutions expect us to be at the forefront of developments, acting as avant-guards. All of this pressure fuels our egos and ambitions; ethics becomes of little account .
Can an ambitious scientist be an ethical scientist? Can ethics coexist with ambition and competition? Considering the enormous potential of AI, where will such acquisitive science lead us as a society?
Disclaimer: This article expresses my assessments as a scientist, and not the views of my present or former employers or any other organization.
References
[1] Grossberg, Michael. History and the disciplining of plagiarism. Originality, imitation, and plagiarism: Teaching writing in the digital age (2008): 159–172.
[2] Pappas, Theodore. “Plagiarism, Culture, and the Future of the Academy.” Humanitas 6, no. 2 (1993): 66–80.
[3] Oxford University Website, Plagiarism,https://tinyurl.com/ynd7z7m4 , visited on June 12, 2023.
[4] Tizhoosh, H.R., The Surge of Sensationalist COVID-19 AI Research, News Medical, May 13, 2020, https://tinyurl.com/2p923jnm
[5] Tizhoosh, H.R., and Jennifer Fratesi. COVID-19, AI enthusiasts, and toy datasets: radiology without radiologists. European Radiology 31, no. 5 (2021): 3553–3554.
[6] Foltýnek, Tomáš, Norman Meuschke, and Bela Gipp. Academic plagiarism detection: a systematic literature review. ACM Computing Surveys (CSUR) 52, no. 6 (2019): 1–42.
[7] Laband, David N., and Michael J. Piette. Favoritism versus search for good papers: Empirical evidence regarding the behavior of journal editors, Journal of Political Economy 102.1 (1994): 194–203.
[8] Colleen Flaherty, When Journals Play Favorites, Inside Higher Ed, 2018, URL: https://tinyurl.com/2ktv82af
[9] Reingewertz, Yaniv, and Carmela Lutmar. Academic in-group bias: An empirical examination of the link between author and journal affiliation, Journal of Informetrics 12.1 (2018): 74–86.
[10] Ipsitaa Khullar, Combatting the privilege of attending elite institutions, London School of Economics and Political Science, 2022, URL: https://bit.ly/3MihJ18
[11] The Economist, Why do American universities favour the children of alumni? 2021, URL: https://tinyurl.com/59w6a6yj
[12] Colleen Flaherty, Publishing’s Prestige Bias, Inside Higher Ed, 2018, URL: https://tinyurl.com/bdepj2wt
[13] Retraction Watch, Controversial AI expert admits to plagiarism, blames hectic schedule, URL: https://tinyurl.com/59s9enw3, visited on June 13, 2023
[14 ] Kalra, Shivam, Hamid R. Tizhoosh, Charles Choi, Sultaan Shah, Phedias Diamandis, Clinton JV Campbell, and Liron Pantanowitz. “Yottixel–an image search engine for large archives of histopathology whole slide images.” Medical Image Analysis 65 (2020): 101757.
[15] Chen, Chengkuan, Ming Y. Lu, Drew FK Williamson, Tiffany Y. Chen, Andrew J. Schaumberg, and Faisal Mahmood. “Fast and scalable search of whole-slide images via self-supervised deep learning.” Nature Biomedical Engineering 6, no. 12 (2022): 1420–1434.
[16] Sikaroudi et al., Comments on “Fast and scalable search of whole-slide images via self-supervised deep learning”, arXiv:2304.08297, https://doi.org/10.48550/arXiv.2304.08297