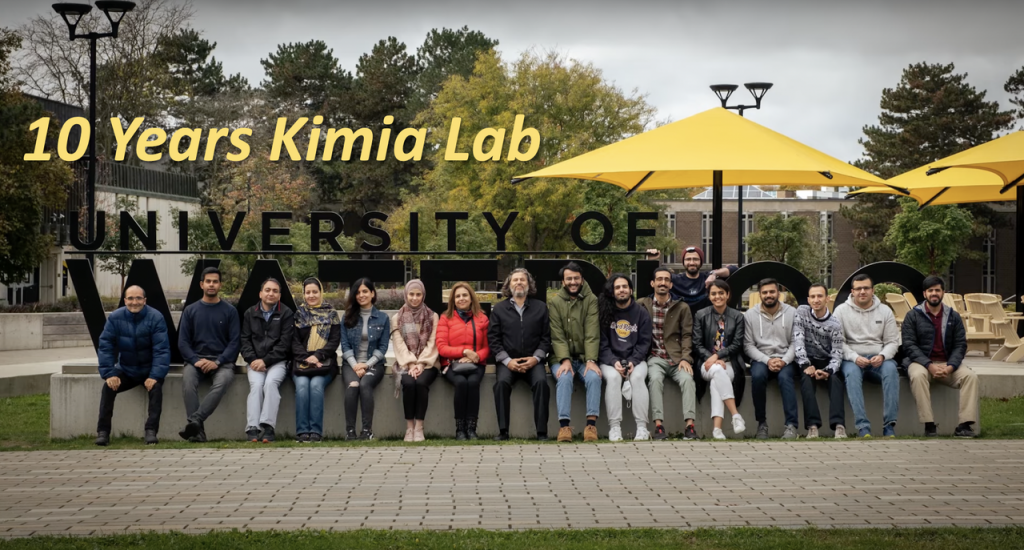
On December 22, 2023, I closed down Kimia Lab at the University of Waterloo. The past ten years have been the most turbulent and most rewarding years in my academic career, and Kimia Lab was in the center of it.
Back in 2012, despite having invested five years of significant effort in the venture, I closed down my AI startup, Segasist Technologies, and resumed my academic routine. Finally, I was able to reduce my workload from 70 hours per week to a more conventional schedule, as I no longer needed to juggle two demanding jobs simultaneously. But, the aftermath of an unsuccessful startup lingered, leaving a lasting and bitter impression – a reminder of defeat after one’s vision failed to materialize.
Working in the realm of academia, such as in a university setting, can quite often feel like an ungrateful job. On the surface, the atmosphere appears to be politically charged, but in essence, it centers around power dynamics and cliques of professors akin to an “old boys’ network”, who tightly control organizational power to influence hiring and, consequently, the allocation of resources. I never aligned myself with any specific group, primarily because I resisted adhering to the concept of “continuity,” a code word for resisting necessary changes at some universities.
Throughout my entrepreneurial activities from 2007 to 2012, when I served as the CTO and CEO of Segasist, I had continued my role as a professor, fulfilling my teaching and research duties to the best of my ability. In fact, my performance exceeded the average benchmark, earning me twice the so-called performance award, though I never placed much value on these internal accolades.
When I returned to my academic routine, the resentment towards me was palpable. It became clear to me that my academic unit did not appreciate my entrepreneurial endeavors. They had always harbored a dislike for me, and my external commercialization activities provided them with a pretext to justify their constant efforts to exclude me. It didn’t matter that the university had a significant stake in the company, and a university administrator sat on the board of my company. It also didn’t matter that my lectures remained as popular as ever, and none of my students had ever complained about potentially being neglected.
I love teaching; the university was merely a framework for me to provide access to students, never the ultimate goal.
In 2012, I found myself burdened not only by the unfortunate legacy of an unsuccessful startup (a transgression for which granting agencies in Canada did not forgive me for several years) but also devoid of research funds, graduate students, a plan, and even a dedicated space for conducting research. Neglecting publications for 4-5 years, I literally faced a survival challenge as a professor.
Determined to turn the tide, I began reaching out to clinicians and radiologists, leveraging my expertise in using AI for medical image analysis—an aspect that had not gained much traction in 2007. My goal was to delve into a new realm of research, seeking a pressing, real-world problem. With no graduate students to supervise, I immersed myself in reading 10 papers a day. The sole beacon in my professional life was teaching; when instructing on topics like data structures, algorithms, computer vision, medical imaging, and machine intelligence, I could momentarily forget the challenges and hostilities around me, leaving the classroom both exhausted and invigorated. Teaching became my lifeline in 2012.
After months of extensive reading and consultations with medical experts, I felt like I had struck gold when I encountered papers on content-based image retrieval, specifically image search. Through numerous discussions with physicians and radiologists, I learned that, most of the time, crucial evidence was missing to support and substantiate medical decisions. The realization struck me— the evidence resided in the archives, within the records of patients who had been diagnosed and treated. We possessed comprehensive information about them, yet we weren’t retrieving this valuable evidence through systematic searches.
It took me some time to identify the related nuances and challenges of how to embed semantic gaps, speed, storage, and the concept of divide and conquer into image search frameworks in medicine.
With no funds to hire students, I delved into coding. Downloading some toy datasets, I began testing ideas. Though I lacked research funds to pay graduate students, I sought out talented students with scholarships. I managed to recruit three self-funded students. Faced with no research space (having unsuccessfully asked my unit in the past), I acquired some old desks from surplus, placed them in my own office, and thus, the seeds of Kimia Lab were sown in 2012, although the lab itself did not exist yet.
Fast forward to 2013, in an old university building where sarcastic comments and curious glances from passersby were the norm (we had to keep the door open; the room was quite small for a professor and three students), we pressed forward. Despite these challenges, my students and I diligently worked on exploring ideas for image search. Papers began to be produced, and it dawned on me that the concept of image search was far grander than my makeshift lab with surplus desks could accommodate—I needed to establish a proper research lab.
In September 2013, as I toyed with the name “Laboratory for Inference in Medical Image Processing,” I realized that the acronym would be IMIP, lacking expressiveness of a unique acronym. While experimenting with different words, the solution appeared before me: “kimia,” the root word for chemistry, steeped in the history of alchemy. The act of searching for images would be akin to transforming data into gold, symbolizing reliable decisions. After some experimentation, the name crystallized: Laboratory of Knowledge Inference in Medical Image Analysis, or simply Kimia Lab.
While a name and historical identity are important, substance is crucial for a research lab. This involves securing a budget to assemble a research team and conduct meaningful research. I applied to various agencies and venues, securing one or two small grants, allowing me to send my students to conferences. I also expanded collaborations and engaged with numerous companies. Unfortunately, Canada lacks a generous granting landscape, and being at a university without a medical school posed a real obstacle.
Around 2014, I found myself increasingly drawn towards pathology. While radiology typically offers an educated guess regarding medical images, pathology serves as the gold standard for many diseases. The visual examination of cellular structures in tissues under the microscope is the definitive method for diagnosis. The heightened responsibility, at least in my perception, appealed to me, recognizing that working on color microscopic images seemed more demanding than analyzing grayscale x-rays and MRIs.
In 2015, at the ICIP in Quebec City, I introduced the concept of “barcodes” for medical images —a straightforward approach to encode the approximate the first derivative of image features. Later, Kimia Lab elevated this concept to master pathology images. Over the next 2-3 years, we generated substantial results, primarily for radiology, showcasing how barcodes could be utilized to tag, annotate, and index medical images. My university’s Office of Research, the sole unit consistently supporting my research, found it “intriguing” and filed a patent for the concept. Scott Inwood, one of my colleagues in WatCo (Waterloo Commercialization office), has consistently been an avid supporter of impactful research, to whom I am greatly indebted.
In 2017, after four years of Kimia Lab, despite our publications, we recognized a need to amplify our presence in computational pathology. A significant obstacle was the shortage of personnel to address the issues we identified, and Kimia Lab required financial support. So, in the summer of 2017, I dedicated almost two months to crafting a substantial application seeking assistance from the Ontario Government. The target program was the Ontario Research Fund – Research Excellence (ORF-RE). To qualify, I had to secure both industrial and clinical partners.
An early supporter was Dr. Liron Pantanowitz from UPMC, a pioneer in digital pathology, whose advice and international reputation significantly bolstered our application. Additionally, we had initiated collaboration with Dr. Clinton JV Campbell, a dynamic hematopathologist from Halifax (later at McMaster University), who provided mentorship during our early foray into computational pathology. Numerous others lent their support. Aaron Pereira from our Office of Research (now Managing Director at WatSPEED) dedicated weeks to assisting me through the intricate ORF-RE application process. His support was invaluable, and without him, the application submission would have been immensely challenging. Cecilia Jenkins also played a crucial role in refining the application package.
Simultaneously, we further developed the concept of transforming images into barcodes, publishing extensively and introducing KimiaPath24, a small dataset showcasing the vast pattern diversity of human tissue. With numerous papers on using CNNs, autoencoders, and “deep barcodes” for image search between 2015 and 2018, Kimia Lab had solidified its position as a prominent force in computational pathology and medical image analysis.
On a cold Friday morning, April 20, 2018, I entered my office as usual to start the day when my phone rang. Dr. Charmaine Dean, the vice president of research, was on the other end, delivering the news that my ORF-RE application had been funded with a $3.2 million grant to investigate AI models for image search in histopathology. For those not familiar with the Canadian context, this was a substantial amount, signifying a pivotal moment for Canadian researchers. It marked the day my research finally received the support it had needed after 17 years of waiting and countless attempts.
Kimia Lab experienced rapid growth; we now possessed the intellectual and coding capacity to tackle our primary goal: creating an image search engine for histopathology. In contrast to radiology, pathology images are both colorful and enormous, presenting ongoing processing challenges. We developed various solutions to divide and conquer whole slide images—the digitized biopsy samples on glass slides. From customizing the dictionary approach to creating novel algorithms, we experimented with numerous ideas.
Enter Yottixel, a portmanteau for “one yotta pixel,” a sophisticated image search engine. Although published a year after Google’s SMILY, Yottixel surpassed it on many levels as a more advanced search solution. Yottixel introduced the concept of “mosaic,” a multistage unsupervised framework to break down gigapixel images into a small, representative set of manageable sub-images called patches. Additionally, Yottixel pioneered the revolutionary notion of a “bunch of barcodes” to binarize the deep features of the mosaic of a tissue image. To this day, Yottixel remains the best search engine for histopathology, extensively validated on the entire 33,000 images of NIH’s repository, TCGA (The Cancer Genome Atlas).
Yottixel’s innovation and success were marred by an audacious attempt from a group to cleverly repackage it under an altered guise but dilettantly destroy its simplicity and efficiency. The computational pathology community requires time to recognize the extent of harm that such dishonest actions can inflict on the productivity, trustworthiness and authenticity of scholarly work within this field.
With more than 100 papers, Kimia Lab proposed numerous ideas and algorithms for image search, covering stain normalization, magnification recognition, federated learning, and image representation. Over 60 papers contain the words “search,” “matching,” and “retrieval” in the title. Worldwide, no other research group has focused as intensely on image search as Kimia Lab.
In May 2021, we published the paper on KimiaNet, the first CNN fully trained with all diagnostic images of the TCGA. The design and training of KimiaNet had commenced in late summer 2019. Between August and December of 2019, all Kimia Lab members dedicated their efforts to data preparation and the training of KimiaNet. To date, KimiaNet excels in providing high-quality embeddings for tissue images, surpassing foundation models.
In accordance with our mandate from the Ontario Government, we commercialized Yottixel through an Ontario-based company. Collaborating with corporations posed challenges, especially when they mistakenly believed a modest financial contribution entitled them to ownership of all our ideas. What I find particularly abhorrent, aside from academic ignorance, is dealing with parochial business owners lacking an understanding of academic freedom. Consequently, I had to sever ties with some partners, a drastic measure causing headaches and further resentment from my academic institution.
Amidst these challenges, we sought to elevate Kimia’s research, coinciding with the onset of the COVID-19 pandemic. While working on a WHO project to search in their breast cancer taxonomy, we encountered a significant obstacle. Despite our capabilities, we were hampered by a lack of data, a recurring issue exacerbated by the constraints of working at a university without a medical school.
In 2020, after a year of renovations, Kimia Lab finally relocated to its new space amid the pandemic. The entire lab was custom-designed, spacious, well-equipped, and adorned with plants everywhere. Kimia Lab, once just a dream, had become a tangible reality. Accompanied with all scientific contributions of the lab, this was the pinnacle of Kimia Lab for all of us.
Shortly after, I decided to leave Canada. It was not an easy decision, and in truth, it wasn’t a decision at all; I simply knew it was time to move on. It was a bittersweet situation. I cherished my students, relished my time in the lab, but as the Germans say, “Man soll aufhören, wenn es am schönsten ist” (One should stop when it’s most beautiful). Conversations with several hospitals revealed Mayo Clinic’s interest in my ideas. Mayo Clinic generously supported the transition of Kimia Lab’s operations. Additionally, Mayo provided a substantial startup grant, enabling me to bring any Kimia students with me. I extended the invitation to all Kimia students, and while some seized the opportunity, others pursued different plans.
The last three Ph.D. students at Kimia Lab are set to graduate in January 2024. After a decade of research, marked by numerous exciting challenges, Kimia Lab stands as an internationally recognized research entity in AI and computational pathology. It is now time to close this chapter and move forward.
Many students, visiting scholars, PDfs and and other team members have contributed to the success of Kimia Lab:
Shivam Kalra, Aditya Sriram, Mitra Seyedahmad, Abtin Riasatian, Areej Alsaafin, Abubakr Shafique, Amir Safarpoor, Sobhan Hemati, Antonio Sze-To, Maryam Hosseini, Soheila Nadalian, Sobhan Shafiei, Danial Maleki, Milad Sikaroudi, Morteza Babaie, RICARDO GONZALEZ, Mohammed Adnan, Mehdi Afshari, Parsa Ashrafi, Zehra Çamlıca, Wafa CHENNI, Kimia Hemmatirad, Habib Herbi, Amin Khatami, Meghana Dinesh Kumar, Maral (Mary) Rasooli, Mahjabin Sajadi, Manit Zaveri, and last but not least Shujin Zhu.
Kimia Lab at the University of Waterloo has ceased to exist. However, the legacy of Kimia Lab endures. Its vision transcends geographical and national confines; it is a pioneering pursuit in multimodal search within medicine aimed at establishing computational consensus.
Further developments forthcoming….
Hamid R. Tizhoosh – December 31, 2023
The perspectives conveyed within this article reflect solely the viewpoints of the author and are not indicative of the stances held by his past or present employers.
References
Yottixel search engine and its validation
KimiaNet paper and the network