Dec 2016
Searching for similar images within vast archives of digital images presents challenges in various domains, including medical image retrieval. While text-based image search is commonly used, it has limitations due to incomplete or inadequate annotations. Content-based image retrieval (CBIR) using non-textual attributes, such as features, has gained popularity. Among recent binary descriptors, Radon barcodes have emerged as a promising option. This paper proposes a new encoding scheme, MinMax Radon barcodes, to enhance the performance of Radon barcodes for medical image retrieval.
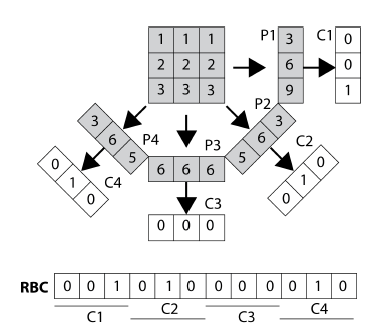
P4) are binarized to create code fragments C1 to C4. Putting all code fragments
together delivers the barcode RBC.
Content-based medical image retrieval has been widely researched, and various approaches, including IRMA dataset-based techniques, have been proposed. Radon transform, commonly used for 3D object capture, has found applications in various fields. Radon composite features (RCFs), histogram of Radon transform (HRT), and other Radon-based techniques have been explored.
Radon barcodes were introduced recently as a method for capturing image content. By projecting images along multiple angles, barcode-like representations of the projections can be formed. The conventional approach involves binarizing projections using local thresholds, but this may lose valuable information. The proposed Radon barcodes are obtained by examining extreme values of projection curvature, providing more expressive shape characteristics.
MinMax Radon barcodes are derived from Radon barcodes using a more sophisticated thresholding method. Instead of simple local thresholds, MinMax Radon barcodes capture the transition between local extrema, making the barcode more descriptive and unique.
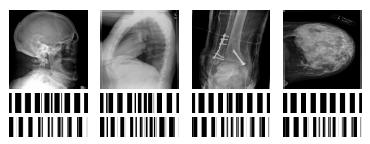
barcodes) for four sample images from IRMA dataset. Images were resized
to 6464 and projected at 8 angles.
The proposed MinMax Radon barcodes were evaluated using the IRMA dataset, containing over 14,000 x-ray images classified into 193 classes. Retrieval performance was measured using Hamming distance, with comparisons made against traditional Radon barcodes and other methods like SURF and BRISK.
The IRMA dataset was used for testing and training purposes. It consists of x-ray images categorized into 193 classes, with IRMA codes representing their annotations.
The error between testing and retrieved images was computed using the IRMA code error calculation formula.
The experiments demonstrated that MinMax Radon barcodes outperformed traditional Radon barcodes, achieving a 15% reduction in retrieval error. Moreover, when compared to SURF and BRISK, MinMax Radon barcodes exhibited higher accuracy, reliability, and faster processing times.
MinMax Radon barcodes offer a more effective and efficient approach to medical image retrieval compared to traditional Radon barcodes, SURF, and BRISK. Their potential for medical applications is significant, and further investigation into optimal projection angles and other encoding schemes may enhance their performance even more.
Additional details: MinMax Radon Barcodes for Medical Image Retrieval