Dec 2016
The proliferation of medical imaging has led to the creation of large image databases and picture archiving and communication systems (PACS) in modern healthcare. To efficiently search these databases, there is a need for accurate and effective methods beyond text-based annotations. Manual annotations by medical experts are time-consuming and limited in describing complex image content. Content-based image retrieval (CBIR) using visual features such as color, texture, and shape addresses these limitations. This paper focuses on the development of Radon-Gabor barcodes, specifically Guided-Radon of Gabor Barcodes (GRGBCs) and Gabor-of-Radon-Image Barcodes (GRIBCs), for extracting shape-texture information from medical images.
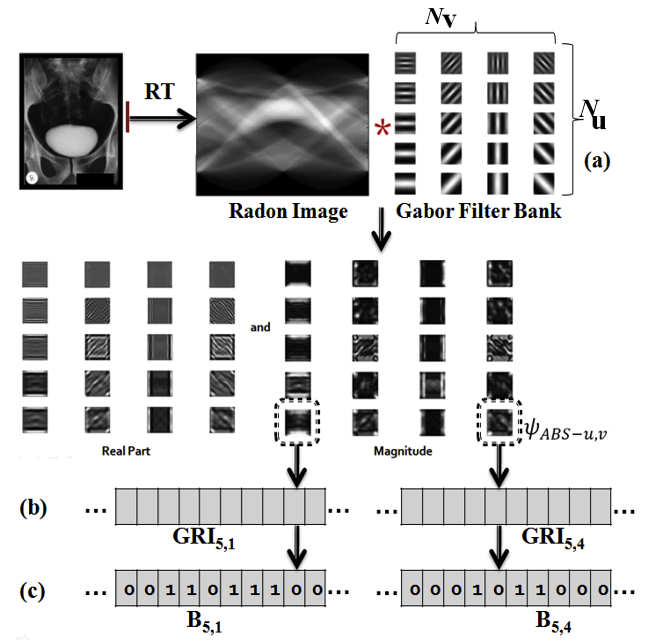
Both Radon and Gabor Transforms are powerful techniques extensively studied in literature. Radon transform captures shape-based details, while Gabor filters excel at extracting texture features robust to geometric variations. By combining Radon and Gabor features, a more comprehensive representation of image content can be achieved, leading to improved retrieval accuracy.
Radon transform captures image features by projecting intensity values along radial lines at specific angles. Barcode annotations are generated by thresholding these projections, resulting in a barcode representation of the image.
Gabor Transform: Gabor filters, based on a Gaussian kernel modulated by a complex sinusoidal wave, provide a multi-resolution analysis of images. They are effective in extracting texture features and are robust to various transformations and disturbances.
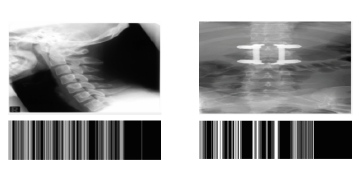
This section introduces two barcode methods: Gabor of Radon Barcodes (GRIBCs) and Guided Radon-Gabor Barcodes (GRGBCs).
- GRIBCs: These barcodes involve applying Radon transform to the image and then applying Gabor filters to the resulting Radon image. Binarization of the feature vectors generates GRIBCs, which provide shape-texture information.
- GRGBCs: This approach combines Radon projections with Gabor filtered responses. Radon projections are applied perpendicular to the parallel stripes in each Gabor filter, resulting in guided Radon-of-Gabor features. Binarization of these features generates GRGBCs.
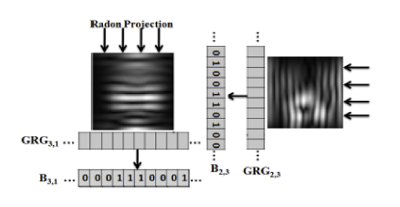
to the direction of stripes in each Gabor filter in GFB.
The experiments were conducted using the IRMA Dataset, which consists of over 14,000 x-ray images with annotated IRMA codes. The performance of the proposed barcodes was evaluated using error measurement scores that account for correct classifications, unspecified codes, and penalties for wrong decisions at different hierarchy levels.
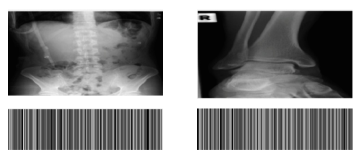
By employing Radon-Gabor barcodes, the retrieval accuracy of medical image databases can be significantly enhanced, providing more comprehensive information about shape and texture features. The experiments conducted on the IRMA Dataset demonstrate the effectiveness of the proposed barcodes for medical image retrieval.
Additional details: Radon-Gabor Barcodes for Medical Image Retrieval